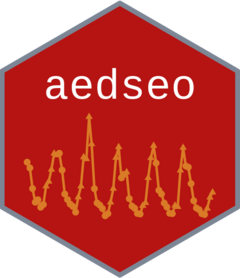
Fits weighted observations to distribution and returns percentiles
Source:R/fit_percentiles.R
fit_percentiles.Rd
This function estimates the percentiles of weighted time series observations. The output contains the percentiles from the fitted distribution.
Arguments
- weighted_observations
A tibble containing two columns of length n;
observation
, which contains the data points, andweight
, which is the importance assigned to the observation. Higher weights indicate that an observation has more influence on the model outcome, while lower weights reduce its impact.- conf_levels
A numeric vector specifying the confidence levels for parameter estimates. The values have to be unique and in ascending order, that is the lowest level is first and highest level is last.
- family
A character string specifying the family for modeling
- optim_method
A character string specifying the method to be used in the optimisation. Lookup
?optim::stats
for details about methods. If using the exp family it is recommended to use Brent as it is a one-dimensional optimisation.- lower_optim
A numeric value for the optimisation.
- upper_optim
A numeric value for the optimisation.
Value
A list containing:
'conf_levels': The conf_levels chosen to fit the percentiles.
'percentiles': The percentile results from the fit.
'par': The fit parameters for the chosen family.
par_1:
For 'weibull': Shape parameter (k).
For 'lnorm': Mean of the log-transformed observations.
For 'exp': Rate parameter (rate).
'par_2':
For 'weibull': Scale parameter (scale).
For 'lnorm': Standard deviation of the log-transformed observations.
For 'exp': Not applicable (set to NA).
'obj_value': The value of the objective function - (negative log-likelihood), which represent the minimized objective function value from the optimisation. Smaller value equals better optimisation.
'converged': Logical. TRUE if the optimisation converged.
'family': The distribution family used for the optimization.
'weibull': Uses the Weibull distribution for fitting.
'lnorm': Uses the Log-normal distribution for fitting.
'exp': Uses the Exponential distribution for fitting.
Examples
# Create three seasons with random observations
obs <- 10
season <- c("2018/2019", "2019/2020", "2020/2021")
season_num_rev <- rev(seq(from = 1, to = length(season)))
observations <- rep(stats::rnorm(10, obs), length(season))
# Add into a tibble with decreasing weight for older seasons
data_input <- tibble::tibble(
observation = observations,
weight = 0.8^rep(season_num_rev, each = obs)
)
# Use the model
fit_percentiles(
weighted_observations = data_input,
conf_levels = c(0.50, 0.90, 0.95),
family= "weibull"
)
#> $conf_levels
#> [1] 0.50 0.90 0.95
#>
#> $values
#> [1] 10.30143 11.17685 11.37846
#>
#> $par
#> [1] 14.71951 10.56116
#>
#> $obj_value
#> [1] 22.71257
#>
#> $converged
#> [1] TRUE
#>
#> $family
#> [1] "weibull"
#>